RESEARCH ABSTRACT
Tree species classification is an essential aspect of sustainable forest management. Annual tree harvest, which happens in Valldaura Labs, plays a key role in the process of turning back the pioneering ecosystem, dominated by Aleppo pine (lat.Pinus halepensis) to its primary species (Holly oak; lat. Quercus ilex).
This research aims to provide a tool to classify forest tree species through high resolution RGB images captured with an unmanned aerial vehicle (UAV) and a standard user camera, in order to control and document tree population. Using Deep Learning technology for object detection and CNN (Convolutional Neural Network) classification enables us to find and identify specific tree species (such as invasive aleppo pine) which allows to monitor forest dynamics and evolution.
The project was divided into phases, where the collection of data sets demanded for training the CNN model was conducted. In order to test the Machine learning training algorithm the process was given a ”Phase 0”, where pictures of leaves collected from Pine and Oak tree were photographed, identified and sorted into two classes to later on train the model and run the first test of object detection model.
METODOLOGY
Figure 1. Research plan ”Phase I”
Research process was divided into phases:
Phase I
———- DATA SET – In order to train the neural network model for tree recognition data set must be prepared:
I. RGB sets of images for each species – many pictures of the same species, including different seasonal features. Flights should be executed in various weather/seasonal conditions, minimally in the summer and autumn season. Pictures collected using an UAV, each flight was conducted on 17m altitude.
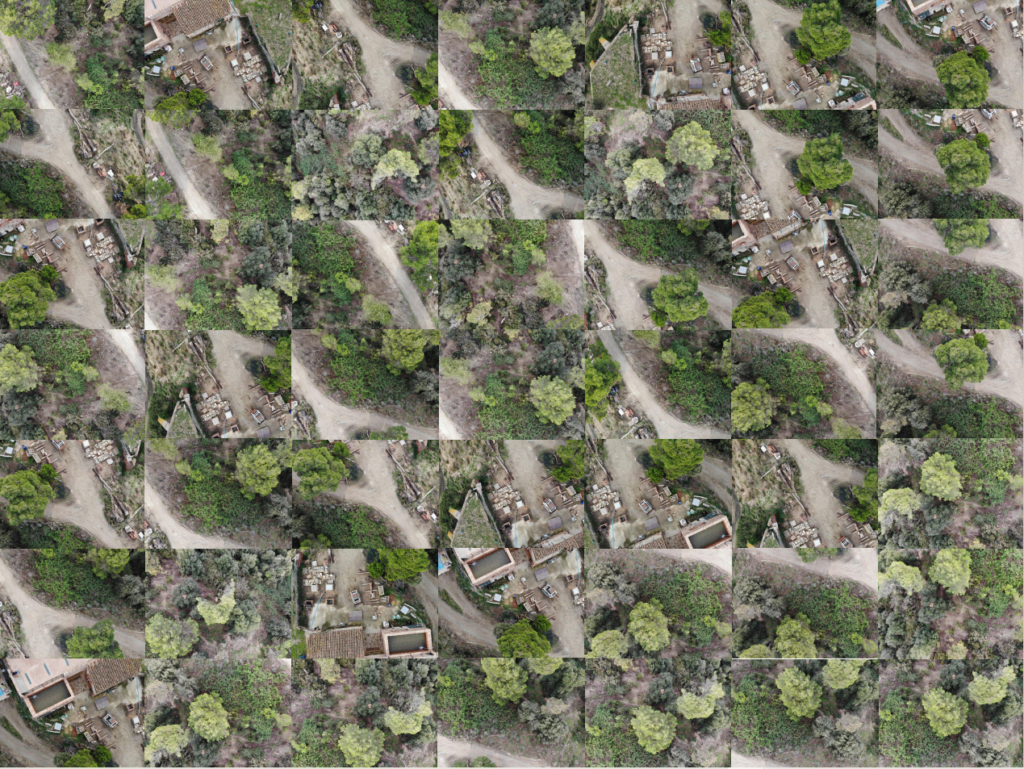
Source: Agnieszka Szklarczyk
Figure 2. Set of images taken with an UAV
II. Digital Surface Model – the photographs were taken with an overlay of 80% and later using their geolocation information connected into an orthomap, on top of Google Maps. This was done using an Open-Source tool WebODM Lighting.
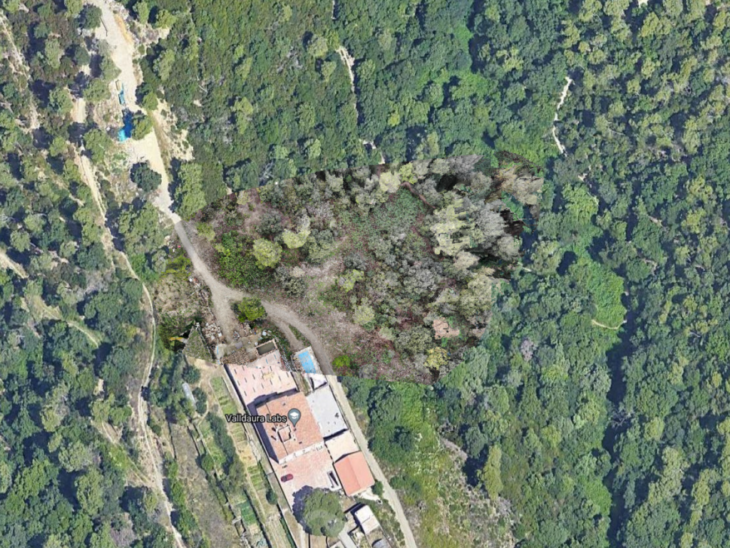
Source: Agnieszka Szklarczyk
Figure 3. Orthomap
III. Tree crown delineation, identification and labeling – selected area of the map set as an input for the Object Recognition model, cutout and labelled images of tree crowns as an output.
Phase ”0”
———- LOAD DATA – To construct a model for Object Identification a publicly available package Teachablemachine for a deep learning framework was used.
This phase was a step down in scale of the Data type. Testing the Object Recognition model on pictures of leaves allowed for operating smaller resolution and faster training processing. This decision was taken to test the tool and later proceed with the same method, using the delineated crown images.
Figure 4. Selected shots from each Data Set
Phase III
———- MAKING PREDICTIONS – Testing the model and its accuracy.
The model was trained on the base of two libraries (sets) contained of 873 photos for the Oak and 902 for Pine. Each library included 3 or more different leaves, representing various stages, which can be encountered in different seasons (green – summer and dry, orange – autumn) of the year.
This video presents how the model reacts and recognized the object exposed to the camera.
Recognition rate reached 91% at the lowest for Oak leaf and 88% for Pine.
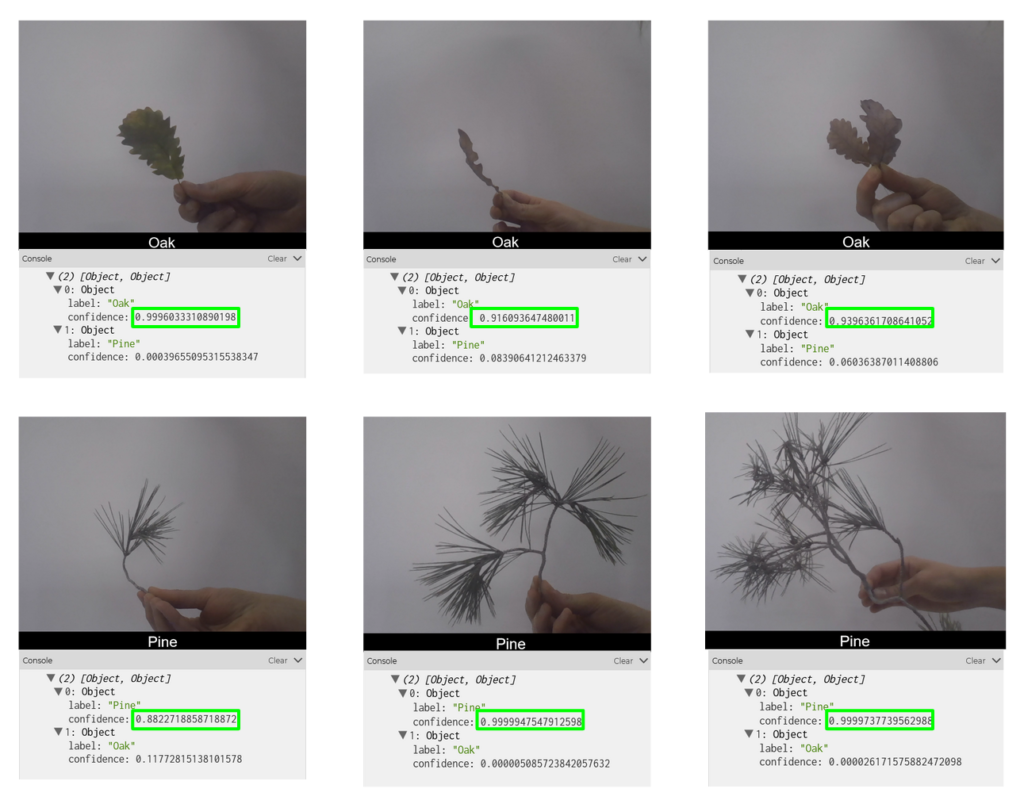
Source: Agnieszka Szklarczyk
CONCLUSION
This study was conducted to investigate the ability of Machine Vision system, which combines UAV RGB images and deep learning, to classify individual trees into several tree types and identify specific tree species.
The drone flying campaigns reviled that for good quality photographs it is important to mind the altitude of the sun and the speed of the wind. Beyond that, photographs capturing characteristic markers, like road, building etc., help for better alignment of the final orthomap.
Regarding the Phase ”0” and testing of the Object Recognition algorithm, the highest number and diversity of photographs loaded into each class, the better identification accuracy can be reached.
Reading the Forest is a project of IAAC, the Institute for Advanced Architecture of Catalonia, developed in the Master in Advanced Ecological Buildings and Biocities (MABB01) 2021/22 by student: Agnieszka Szklarczyk; faculty: Kya Kerner